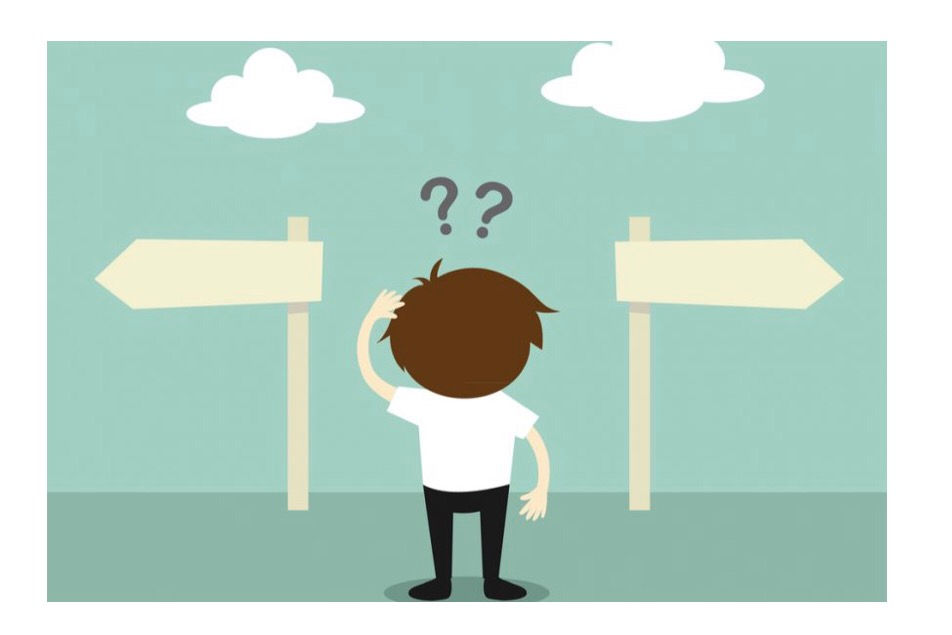
Crescent City, California
June 11, 2021
by Justin Fox (not a relative as far as I know)
From HBR (May 2015)
When we make decisions, we make mistakes. We all know this from personal experience, of course. But just in case we didn’t, a seemingly unending stream of experimental evidence in recent years has documented the human penchant for error. This line of research—dubbed heuristics and biases, although you may be more familiar with its offshoot, behavioral economics—has become the dominant academic approach to understanding decisions. Its practitioners have had a major influence on business, government, and financial markets. Their books—Predictably Irrational; Thinking, Fast and Slow; and Nudge, to name three of the most important—have suffused popular culture.
So far, so good. This research has been enormously informative and valuable. Our world, and our understanding of decision making, would be much poorer without it.
It is not, however, the only useful way to think about making decisions. Even if you restrict your view to the academic discussion, there are three distinct schools of thought. Although heuristics and biases is currently dominant, for the past half century it has interacted with and sometimes battled with the other two, one of which has a formal name—decision analysis—and the other of which can perhaps best be characterized as demonstrating that we humans aren’t as dumb as we look.
Adherents of the three schools have engaged in fierce debates, and although things have settled down lately, major differences persist. This isn’t like David Lodge’s aphorism about academic politics being so vicious because the stakes are so small. Decision making is important, and decision scholars have had real influence.
This article briefly tells the story of where the different streams arose and how they have interacted, beginning with the explosion of interest in the field during and after World War II (for a longer view, see “A Brief History of Decision Making,” by Leigh Buchanan and Andrew O’Connell, HBR, January 2006). The goal is to make you a more informed consumer of decision advice—which just might make you a better decision maker.
The Rational Revolution
During World War II statisticians and others who knew their way around probabilities (mathematicians, physicists, economists) played an unprecedented and crucial role in the Allied effort. They used analytical means—known as operational research in the UK and operations research on this side of the Atlantic—to improve quality control in manufacturing, route ships more safely across the ocean, figure out how many pieces antiaircraft shells should break into when they exploded, and crack the Germans’ codes.
After the war hopes were high that this logical, statistical approach would transform other fields. One famous product of this ambition was the nuclear doctrine of mutual assured destruction. Another was decision analysis, which in its simplest form amounts to (1) formulating a problem, (2) listing the possible courses of action, and (3) systematically assessing each option. Historical precedents existed—Benjamin Franklin had written in the 1770s of using a “Moral or Prudential Algebra” to compare options and make choices. But by the 1950s there was tremendous interest in developing a standard approach to weighing options in an uncertain future.
Updating Probabilities
The math behind Bayes’ Theorem is simple, even if the application of it often isn’t. Here’s an illustration, adapted from Nate Silver’s brilliantly clear explanation in The Signal and the Noise.
Let’s say that before Sept. 11, 2001, you put the odds that terrorists would crash a plane into a New York skyscraper (x) at 0.005%. After the first plane struck, you figured that the odds of a plane’s hitting if in fact terrorists were attacking Manhattan with it (y) was 100%, and the odds of a plane’s hitting by random chance (z) was 0.008%. Plug those into Bayes’s formula, xy/(xy+z(1-x)), and the probability that terrorists had just flown that plane into the World Trade Center is 38%. Run the calculation for a second plane, using 38% as the initial probability, and you get a probability of 99.99%.
The mathematician John von Neumann, who coined the term mutual assured destruction, helped jump-start research into decision making with his notion of “expected utility.” As outlined in the first chapter of his landmark 1944 book Theory of Games and Economic Behavior, written with the economist Oskar Morgenstern, expected utility is what results from combining imagined events with probabilities. Multiply the likelihood of a result against the gains that would accrue, and you get a number, expected utility, to guide your decisions.
It’s seldom that simple, of course. Von Neumann built his analysis around the game of poker, in which potential gains are easily quantifiable. In lots of life decisions, it’s much harder. And then there are the probabilities: If you’re uncertain, how are you supposed to know what those are?
The winning answer was that there is no one right answer—everybody has to wager a guess—but there is one correct way to revise probabilities as new information comes in. That is what has become known as Bayesian statistics, a revival and advancement of long-dormant ideas (most of them the work not of the English reverend Thomas Bayes but of the French mathematical genius Pierre-Simon Laplace) by a succession of scholars starting in the 1930s. For the purposes of storytelling simplicity I’ll mention just one: Leonard Jimmie Savage, a statistics professor whose 1954 book The Foundations of Statistics laid out the rules for changing one’s probability beliefs in the face of new information.
One early and still-influential product of this way of thinking is the theory of portfolio selection, outlined in 1952 by Savage’s University of Chicago student Harry Markowitz, which advised stock pickers to estimate both the expected return on a stock and the likelihood that their estimate was wrong. Markowitz won a Nobel prize for this in 1990.
The broader field of decision analysis began to come together in 1957, when the mathematician Howard Raiffa arrived at Harvard with a joint appointment in the Business School and the department of statistics. He soon found himself teaching a statistics course for business students with Robert Schlaifer, a classics scholar and fast learner who in the postwar years taught pretty much whatever needed teaching at HBS. The two concluded that the standard statistics fare of regressions and P values wasn’t all that useful to future business leaders, so they adopted a Bayesian approach. Before long what they were teaching was more decision making than statistics. Raiffa’s decision trees, with which students calculated the expected value of the different paths available to them, became a staple at HBS and the other business schools that emulated this approach.
The actual term “decision analysis,” though, was coined by Ronald Howard, an MIT electrical engineer and an expert in statistical processes who had studied with some of the leading figures in wartime operations research at MIT and crossed paths with Raiffa in Cambridge. While visiting Stanford for the 1964–1965 academic year, Howard was asked to apply the new decision-making theories to a nuclear power plant being contemplated at General Electric’s nuclear headquarters, then located in San Jose. He combined expected utility and Bayesian statistics with computer modeling and engineering techniques into what he dubbed decision analysis and some of his followers call West Coast decision analysis, to distinguish it from Raiffa’s approach. Howard and Raiffa were honored as the two founding fathers of the field at its 50th-anniversary celebration last year.
Irrationality’s Revenge
Almost as soon as von Neumann and Morgenstern outlined their theory of expected utility, economists began adopting it not just as a model of rational behavior but as a description of how people actually make decisions. “Economic man” was supposed to be a rational creature; since rationality now included assessing probabilities in a consistent way, economic man could be expected to do that, too. For those who found this a bit unrealistic, Savage and the economist Milton Friedman wrote in 1948, the proper analogy was to an expert billiards player who didn’t know the mathematical formulas governing how one ball would carom off another but “made his shots as if he knew the formulas.”
1950s
The term “heuristic” begins to be used in a positive sense.
Somewhat amazingly, that’s where economists left things for more than 30 years. It wasn’t that they thought everybody made perfect probability calculations; they simply believed that in free markets, rational behavior would usually prevail.
The question of whether people actually make decisions in the ways outlined by von Neumann and Savage was thus left to the psychologists. Ward Edwards was the pioneer, learning about expected utility and Bayesian methods from his Harvard statistics professor and writing a seminal 1954 article titled “The Theory of Decision Making” for a psychology journal. This interest was not immediately embraced by his colleagues—Edwards was dismissed from his first job, at Johns Hopkins, for focusing too much on decision research. But after a stint at an Air Force personnel research center, he landed at the University of Michigan, a burgeoning center of mathematical psychology. Before long he lured Jimmie Savage to Ann Arbor and began designing experiments to measure how well people’s probability judgments followed Savage’s axioms.
A typical Edwards experiment went like this: Subjects were shown two bags of poker chips—one containing 700 red chips and 300 blue chips, and the other the opposite. Subjects took a few chips out of a random bag and then estimated the likelihood that they had the mostly blue bag or the mostly red one.
Say you got eight red chips and four blue ones. What’s the likelihood that you had the predominantly red bag? Most people gave an answer between 70% and 80%. According to Bayes’ Theorem, the likelihood is actually 97%.
Still, the changes in subjects’ probability assessments were “orderly” and in the correct direction, so Edwards concluded in 1968 that people were “conservative information processors”—not perfectly rational according to the rules of decision analysis, but close enough for most purposes.
In 1969 Daniel Kahneman, of the Hebrew University of Jerusalem, invited a colleague who had studied with Edwards at the University of Michigan, Amos Tversky, to address his graduate seminar on the practical applications of psychological research. Tversky told the class about Edwards’s experiments and conclusions. Kahneman, who had not previously focused on decision research, thought Edwards was far too generous in his assessment of people’s information-processing skills, and before long he persuaded Tversky to undertake a joint research project. Starting with a quiz administered to their fellow mathematical psychologists at a conference, the pair conducted experiment after experiment showing that people assessed probabilities and made decisions in ways systematically different from what the decision analysts advised.
“In making predictions and judgments under uncertainty, people do not appear to follow the calculus of chance or the statistical theory of prediction,” they wrote in 1973. “They rely on a limited number of heuristics which sometimes yield reasonable judgments and sometimes lead to severe and systematic errors.”
Heuristics are rules of thumb—decision-making shortcuts. Kahneman and Tversky didn’t think relying on them was always a bad idea, but they focused their work on heuristics that led people astray. Over the years they and their adherents assembled a long list of these decision-making flaws—the availability heuristic, the endowment effect, and so on.
As an academic movement, this was brilliantly successful. Kahneman and Tversky not only attracted a legion of followers in psychology but also inspired a young economist, Richard Thaler, and with help from him and others came to have a bigger impact on the field than any outsider since von Neumann. Kahneman won an economics Nobel in 2002—Tversky had died in 1996 and thus couldn’t share the prize—and the heuristics-and-biases insights relating to money became known as behavioral economics. The search for ways in which humans violate the rules of rationality remains a rich vein of research for scholars in multiple fields.
Rules of Thumb
Heuristics can be useful, but they can also lead to systematic errors. ...
The implications for how to make better decisions, though, are less clear. First-generation decision analysts such as Howard Raiffa and Ward Edwards recognized the flaws described by Kahneman and Tversky as real but thought the focus on them was misplaced and led to a fatalistic view of man as a “cognitive cripple.” Even some heuristics-and-biases researchers agreed. “The bias story is so captivating that it overwhelmed the heuristics story,” says Baruch Fischhoff, a former research assistant of Kahneman and Tversky who has long taught at Carnegie Mellon University. “I often cringe when my work with Amos is credited with demonstrating that human choices are irrational,” Kahneman himself wrote in Thinking, Fast and Slow. “In fact our research only showed that humans are not well described by the rational-agent model.” And so a new set of decision scholars began to examine whether those shortcuts our brains take are actually all that irrational.
When Heuristics Work
That notion wasn’t entirely new. Herbert Simon, originally a political scientist but later a sort of social scientist of all trades (the economists gave him a Nobel in 1978), had begun using the term “heuristic” in a positive sense in the 1950s. Decision makers seldom had the time or mental processing power to follow the optimization process outlined by the decision analysts, he argued, so they “satisficed” by taking shortcuts and going with the first satisfactory course of action rather than continuing to search for the best.
Simon’s “bounded rationality,” as he called it, is often depicted as a precursor to the work of Kahneman and Tversky, but it was different in intent. Whereas they showed how people departed from the rational model for making decisions, Simon disputed that the “rational” model was actually best. In the 1980s others began to join in the argument.
The most argumentative among them was and still is Gerd Gigerenzer, a German psychology professor who also did doctoral studies in statistics. In the early 1980s he spent a life-changing year at the Center for Interdisciplinary Research in the German city of Bielefeld, studying the rise of probability theory in the 17th through 19th centuries with a group of philosophers and historians. One result was a well-regarded history, The Empire of Chance, by Gigerenzer and five others (Gigerenzer’s name was listed first because in keeping with the book’s theme, the authors drew lots). Another was a growing conviction in Gigerenzer’s mind that the Bayesian approach to probability favored by the decision analysts was, although not incorrect, just one of several options.
1968
The psychologist Ward Edwards finds that people are “conservative information processors”—not perfectly rational according to the rules of decision analysis, but close enough for most purposes.
When Gigerenzer began reading Kahneman and Tversky, he says now, he did so “with a different eye than most readers.” He was, first, dubious of some of the results. By tweaking the framing of a question, it is sometimes possible to make apparent cognitive illusions go away. Gigerenzer and several coauthors found, for example, that doctors and patients are far more likely to assess disease risks correctly when statistics are presented as natural frequencies (10 out of every 1,000) rather than as percentages.
But Gigerenzer wasn’t content to leave it at that. During an academic year at Stanford’s Center for Advanced Study in the Behavioral Sciences, in 1989–1990, he gave talks at Stanford (which had become Tversky’s academic home) and UC Berkeley (where Kahneman then taught) fiercely criticizing the heuristics-and-biases research program. His complaint was that the work of Kahneman, Tversky, and their followers documented violations of a model, Bayesian decision analysis, that was itself flawed or at best incomplete. Kahneman encouraged the debate at first, Gigerenzer says, but eventually tired of his challenger’s combative approach. The discussion was later committed to print in a series of journal articles, and after reading through the whole exchange, it’s hard not to share Kahneman’s fatigue.
Gigerenzer is not alone, though, in arguing that we shouldn’t be too quick to dismiss the heuristics, gut feelings, snap judgments, and other methods humans use to make decisions as necessarily inferior to the probability-based verdicts of the decision analysts. Even Kahneman shares this belief to some extent. He sought out a more congenial discussion partner in the psychologist and decision consultant Gary Klein. One of the stars of Malcolm Gladwell’s book Blink, Klein studies how people — firefighters, soldiers, pilots — develop expertise, and he generally sees the process as being a lot more naturalistic and impressionistic than the models of the decision analysts. He and Kahneman have together studied when going with the gut works and concluded that, in Klein’s words, “reliable intuitions need predictable situations with opportunities for learning.”
Are those really the only situations in which heuristics trump decision analysis? Gigerenzer says no, and the experience of the past few years (the global financial crisis, mainly) seems to back him up. When there’s lots of uncertainty, he argues, “you have to simplify in order to be robust. You can’t optimize any more.” In other words, when the probabilities you feed into a decision-making model are unreliable, you might be better off following a rule of thumb. One of Gigerenzer’s favorite examples of this comes from Harry Markowitz, the creator of the decision analysis cousin known as modern portfolio theory, who once let slip that in choosing the funds for his retirement account, he had simply split the money evenly among the options on offer (his allocation for each was 1/N). Subsequent research has shown that this so-called 1/N heuristic isn’t a bad approach at all.
The State of the Art
The Kahneman-Tversky heuristics-and-biases approach has the upper hand right now, both in academia and in the public mind. Aside from its many real virtues, it is the approach best suited to obtaining interesting new experimental results, which are extremely helpful to young professors trying to get tenure. Plus, journalists love writing about it.
Decision analysis hasn’t gone away, however. HBS dropped it as a required course in 1997, but that was in part because many students were already familiar with such core techniques as the decision tree. As a subject of advanced academic research, though, it is confined to a few universities—USC, Duke, Texas A&M, and Stanford, where Ron Howard teaches. It is concentrated in industries, such as oil and gas and pharmaceuticals, in which managers have to make big decisions with long investment horizons and somewhat reliable data. Chevron is almost certainly the most enthusiastic adherent, with 250 decision analysts on staff. Aspects of the field have also enjoyed an informal renaissance among computer scientists and others of a quantitative bent. The presidential election forecasts that made Nate Silver famous were a straightforward application of Bayesian methods.
2002
Daniel Kahneman wins an economics Nobel for his work on heuristics.
Those who argue that rational, optimizing decision making shouldn’t be the ideal are a more scattered lot. Gigerenzer has a big group of researchers at the Max Planck Institute for Human Development, in Berlin. Klein and his allies, chiefly in industry and government rather than academia, gather regularly for Naturalistic Decision Making conferences. Academic decision scholars who aren’t decision analysts mostly belong to the interdisciplinary Society for Judgment and Decision Making, which is dominated by heuristics-and-biases researchers. “It’s still very much us and them, where us is Kahneman-and-Tversky disciples and the rest is Gerd and people who have worked with him,” says Dan Goldstein, a former Gigerenzer student now at Microsoft Research. “It’s still 90 to 10 Kahneman and Tversky.” Then again, Goldstein—a far more diplomatic sort than his mentor—is slated to be the next president of the society.
There seems to be more overlap in practical decision advice than in decision research. The leading business school textbook, Judgment in Managerial Decision Making, by Harvard’s Max Bazerman (and, in later editions, UC Berkeley’s Don Moore), devotes most of its pages to heuristics and biases but is dedicated to the decision analyst Howard Raiffa and concludes with a list of recommendations that begins, “1. Use decision analysis tools.” There’s nothing inconsistent there—the starting point of the whole Kahneman-and-Tversky research project was that decision analysis was the best approach. But other researchers in this tradition, when they try to correct the decision-making errors people make, also find themselves turning to heuristics.
One of the best-known products of heuristics-and-biases research, Richard Thaler and Shlomo Benartzi’s Save More Tomorrow program, replaces the difficult choices workers face when asked how much they want to put aside for retirement with a heuristic—a commitment to automatically bump up one’s contribution with every pay raise—that has led to dramatic increases in saving. A recent field experiment with small-business owners in the Dominican Republic found that teaching them the simple heuristic of keeping separate purses for business and personal life, and moving money from one to the other only once a month, had a much greater impact than conventional financial education. “The big challenge is to know the realm of applications where these heuristics are useful, and where they are useless or even harm people,” says the MIT economist Antoinette Schoar, one of the researchers. “At least from what I’ve seen, we don’t know very well what the boundaries are of where heuristics work.”
This has recently been a major research project for Gigerenzer and his allies—he calls it the study of “ecological rationality.” In environments where uncertainty is high, the number of potential alternatives many, or the sample size small, the group argues, heuristics are likely to outperform more-analytic decision-making approaches. This taxonomy may not catch on—but the sense that smart decision making consists of a mix of rational models, error avoidance, and heuristics seems to be growing.
Other important developments are emerging. Advances in neuroscience could change the decision equation as scientists get a better sense of how the brain makes choices, although that research is in early days. Decisions are increasingly shunted from people to computers, which aren’t subject to the same information-processing limits or biases humans face. But the pioneers of artificial intelligence included both John von Neumann and Herbert Simon, and the field still mixes the former’s decision-analysis tools with the latter’s heuristics. It offers no definitive verdict—yet—on which approach is best.
Making Better Decisions
So, what is the right way to think about making decisions? There are a few easy answers. For big, expensive projects for which reasonably reliable data is available—deciding whether to build an oil refinery, or whether to go to an expensive graduate school, or whether to undergo a medical procedure—the techniques of decision analysis are invaluable. They are also useful in negotiations and group decisions. Those who have used decision analysis for years say they find themselves putting it to work even for fast judgments. The Harvard economist Richard Zeckhauser runs a quick decision tree in his head before deciding how much money to put in a parking meter in Harvard Square. “It sometimes annoys people,” he admits, “but you get good at doing this.”
A firefighter running into a burning building doesn’t have time for even a quick decision tree, yet if he is experienced enough his intuition will often lead him to excellent decisions. Many other fields are similarly conducive to intuition built through years of practice—a minimum of 10,000 hours of deliberate practice to develop true expertise, the psychologist K. Anders Ericsson famously estimated. The fields where this rule best applies tend to be stable. The behavior of tennis balls or violins or even fire won’t suddenly change and render experience invalid.
Management isn’t really one of those fields. It’s a mix of situations that repeat themselves, in which experience-based intuitions are invaluable, and new situations, in which such intuitions are worthless. It involves projects whose risks and potential returns lend themselves to calculations but also includes groundbreaking endeavors for which calculations are likely to mislead. It is perhaps the profession most in need of multiple decision strategies.
Part of the appeal of heuristics-and-biases research is that even if it doesn’t tell you what decision to make, it at least warns you away from ways of thought that are obviously wrong. If being aware of the endowment effect makes you less likely to defend a declining business line rather than invest in a new one, you’ll probably be better off.
Yet overconfidence in one’s judgment or odds of success—near the top of most lists of decision-making flaws—is a trait of many successful leaders. At the very cutting edge of business, it may be that good decision making looks a little like the dynamic between Star Trek’s Captain Kirk and Mr. Spock, with Spock reciting the preposterously long odds of success and Kirk confidently barging ahead, Spock still at his side.
Comentarios